Stop Calling Everything AI, Says Leading Researcher
- By DSAITrends editors
- April 07, 2021
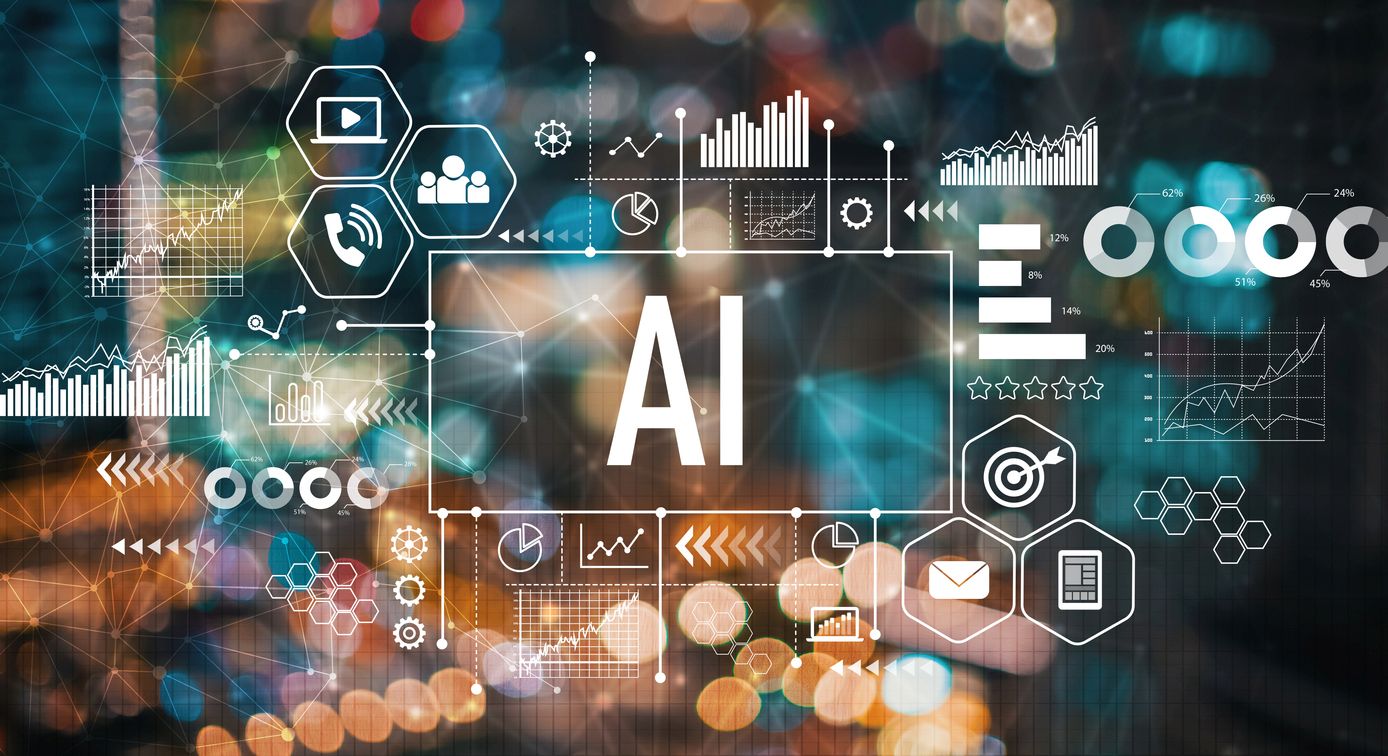
A leading researcher on AI and machine learning (ML) has issued a call to stop dubbing everything as AI in an article published on IEEE Spectrum.
The rationale? While AI systems can show human-level competence in low-level pattern recognition skills, they are merely imitating human intelligence on a cognitive level, says Michael I. Jordan.
Jordan is a professor in the department of electrical engineering and computer science, and the department of statistics, at the University of California, Berkeley.
Considered by some as one of the foremost authorities on AI and ML and credited with transforming unsupervised machine learning from a collection of algorithms to an intellectually coherent field, this was hardly the first time Jordan has put a dampener on AI.
Machine learning
In this case, his frustration stems from the fact that when most people talk about AI, they mean ML.
“People are getting confused about the meaning of AI in discussions of technology trends – that there is some kind of intelligent thought in computers that is responsible for the progress and which is competing with humans,” he said.
In a previous article on Medium titled “AI – The revolution hasn’t happened yet”, Jordan said this of ML: “ML is an algorithmic field that blends ideas from statistics, computer science, and many other disciplines to design algorithms that process data, make predictions, and help make decisions.”
The problem with science-fiction discussions about AI though, is how they distract from a focus on the real problem, says Jordan. In his words, we should be focusing on “building planetary-scale machine learning-based systems that work, deliver value to humans, and do not amplify inequities.”
The human in the machine
For what it’s worth, Jordan does not think computers can match humans in their ability to address real-world situations anytime soon. Instead, he sees machines supporting humans to solve our “most pressing problems”.
And the role of humans in solving these problems is inextricable: “We will need well-thought-out interactions of humans and computers to solve our most pressing problems. We need to understand that the intelligent behavior of large-scale systems arises as much from the interactions among agents as from the intelligence of individual agents.”
Ironic it might be, but it does sound like finding success in AI might be to stop focusing on it, and instead look at ways to make our lives better through the judicious application of data science and ML.
Image credit: iStockphoto/Melpomenem