Empowering the Intelligent Data-Driven Enterprise in the Cloud
- By Paul Mah
- August 26, 2021
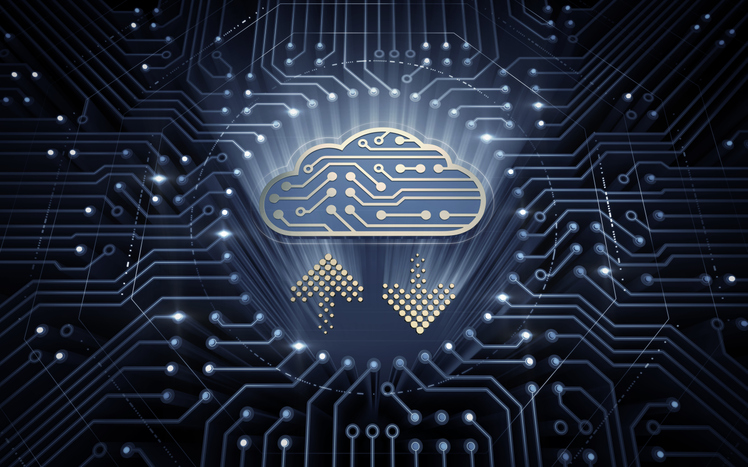
Businesses realize that the cloud offers a lot more than digital infrastructure. Around the world, organizations are turning to the cloud to democratize data access, harness advanced AI and analytics capabilities, and make better data-driven business decisions.
But despite heavy investments to build data repositories, set up advanced database management systems (DBMS), and build large data warehouses on-premises, many enterprises are still challenged with poor business outcomes, observed Anthony Deighton, chief product officer at Tamr.
Deighton was speaking at the “Empowering the intelligent data-driven enterprise in the cloud” event by Tamr and Google Cloud in conjunction with CDOTrends. Attended by top innovation executives, data leaders, and data scientists from Asia Pacific, the virtual panel discussion looked at how forward-looking businesses might kick off the next phase of data transformation.
Why a DataOps strategy makes sense
“Despite this massive [and ongoing] revolution in data, customers still can't get a view of their customers, their suppliers, and the materials they use in their business. Their analytics are out-of-date, or their AI initiatives are using bad data and therefore making bad recommendations. The result is that people don't trust the data in their systems,” said Deighton.
“As much as we've seen a revolution in the data infrastructure space, we're not seeing a better outcome for businesses. To succeed, we need to think about changing the way we work with data,” he explained.
And this is where a DataOps strategy comes into play. A direct play on the popular DevOps strategy for software development, DataOps relies on an automated, process-oriented methodology to improve data quality for data analytics. Deighton thinks the DevOps revolution in software development can be replicated with data through a continuous collaborative approach with best-of-breed systems and the cloud.
“Think of Tamr working in the backend to clean and deliver this centralized master data in the cloud. Offering clean, curated sources to questions such as: Who are my customers? What products have we sold? What vendors do we do business with? What are my sales transactions? And of course, for every one of your [departments], there's a different set of these clean, curated business topics that are relevant to you.”
Data in an intelligent cloud
But won’t an on-premises data infrastructure work just as well? So what benefits does the cloud offer? Deighton outlined two distinct advantages to explain why he considers the cloud the linchpin of the next phase of data transformation.
“You can store infinite amounts of data in the cloud, and you can do that very cost-effectively. It's far less costly to store data in the cloud than it is to try to store it on-premises, in [your own] data lakes,” he said.
“Another really powerful capability of Google Cloud is its highly scalable elastic compute infrastructure. We can leverage its highly elastic compute and the fact that the data is already there. And then we can run our human-guided machine learning algorithms cost-effectively and get on top of that data quickly.”
Andrew Psaltis, the APAC technology practice lead at Google Cloud, drew attention to the synergy between Tamr and Google Cloud.
“You can get data into [Google] BigQuery in different ways, but what you really want is clean, high-quality data. That quality allows you to have confidence in your advanced analytics, machine learning, and to the entire breadth of our analytics and AI platform. We have an entire platform to enable you to collaborate with your data science team; we have the tooling to do so without code, packaged AI solutions, tools for those who prefer to write their code, and everywhere in between.”
Bridging the data silos
A handful of polls were conducted as part of the panel event, which saw participants quizzed about their ongoing data-driven initiatives. When asked about how they are staffing their data science initiatives, the top response by participants (46%) cited having multiple teams across various departments for their data science initiatives.
The rest are split between either having a central team collecting, processing, and analyzing data or a combination of a central team working with multiple project teams across departments.
Deighton observed that multiple work teams typically result in multiple data silos: “Each team has their silo of data. Maybe the team is tied to a specific business unit, a specific product team, or maybe a specific customer sales team.”
The way to break the data barriers is to bring data together in the cloud to give users a view of the data across teams, he says. “And it may sound funny, but sometimes, the way to break the interpersonal barriers is by breaking the data barriers.”
“Your customers don't care how you are organized internally. They want to do business with you, with your company. If you think about it, not from the perspective of the team, but the customer, then you need to put more effort into resolving your data challenges to best serve your customers.”
Making the move
When asked about their big data change initiatives for the next three years, the response was unanimous: Participants want to democratize analytics, build a data culture, and make decisions faster (86%). Unsurprisingly, the top roadblock is that IT takes too long to deliver the systems data scientists need (62%) and the cost of data solutions (31%).
The cloud makes sense, given how it enables better work efficiency, lowers operational expenses, and is inherently secure, said Psaltis. Workers are moving to the cloud, Psaltis noted as he shared an anecdote about an unnamed organization that loaded the cloud with up to a petabyte of data in relatively short order.
This was apparently done without the involvement or knowledge of the IT department. Perhaps it might be better if the move to the cloud is done under more controlled circumstances with the approval and participation of IT, says Psaltis.
Finally, it is imperative that data is cleaned – and kept clean – as it is migrated to the cloud. “Simply moving it into the cloud isn't enough. Without cleaning the data first, you will end up with poor quality, disparate data in the cloud where each application’s data sits within a silo (with more silos than before), making it difficult to make quality business decisions,” summed up Deighton.
Paul Mah is the editor of DSAITrends. A former system administrator, programmer, and IT lecturer, he enjoys writing both code and prose. You can reach him at [email protected].
Image credit: iStockphoto/Artystarty