5 Tips to Level up Your Data Science Team in 2023
- By Paul Mah
- November 27, 2022
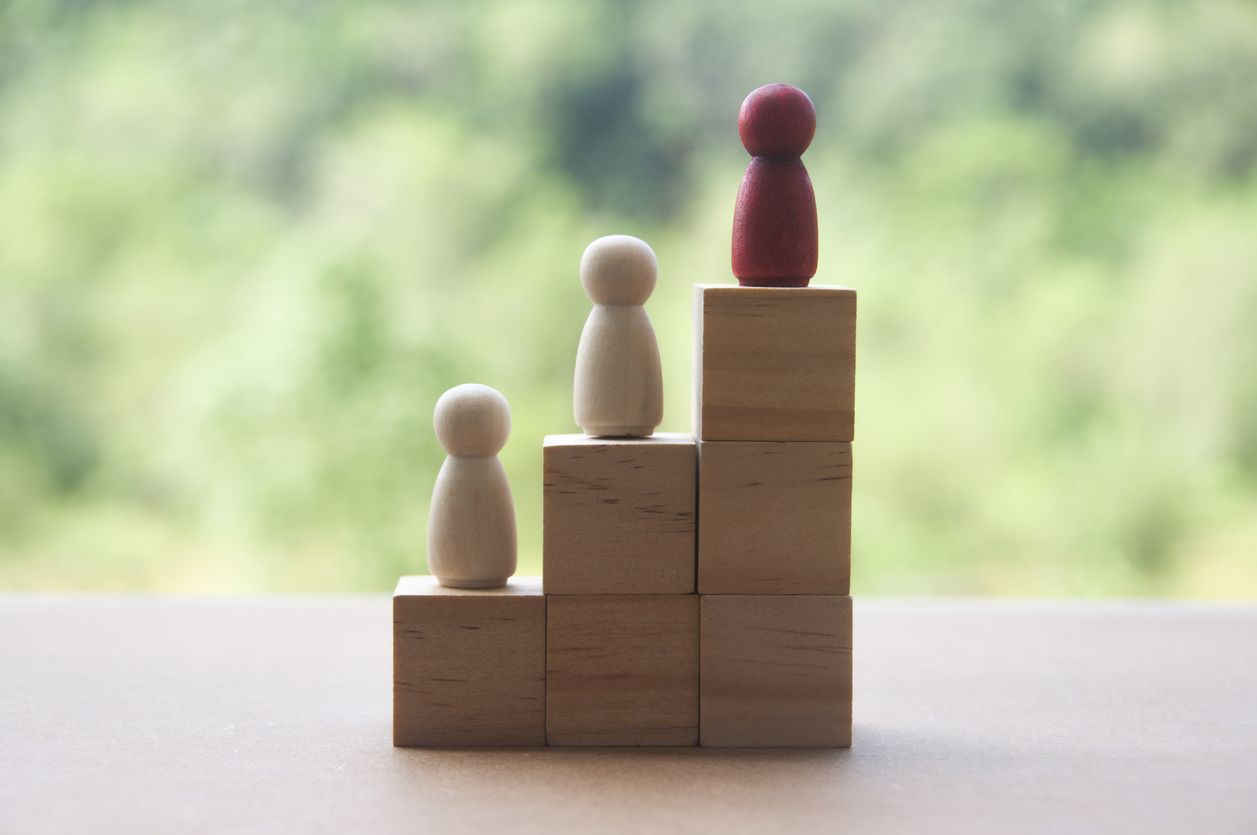
As we come to the end of 2022, it is increasingly clear that data science is no longer the domain of enterprises but that even mid-sized businesses and SMBs are turning to it. With a vast amount of data at their disposal, organizations large and small are turning to data and analytics to gain an advantage over their competitors.
Here are some top data science tips I combed up to help level up your data science in 2023.
Gear up to work in the cloud
With a growing pool of data reaching unmanageable levels, data transformation is getting harder, not easier. Organizations are not just grappling with an increasing deluge of data but legacy systems and various inherited data structures. And the cloud might be the only way for businesses to come out ahead.
Specifically, the cloud makes it possible to centralize and connect the dots between data sources. As noted by Microsoft’s Karthikeyan Rajasekharan, this allows customers to ask better questions, including questions they were not able to ask previously.
The cloud also offers greater access to new tools that might not otherwise be available. For instance, I wrote recently about how a mechanical engineering student trained a Stable Diffusion model by renting GPUs on the cloud-based Vast.ai for a couple of dollars. With the cloud, the sky is the limit.
Data science is now a team sport
The days of a small handful of top data scientists being able to address the entire organization’s needs are over. The breadth and scope of data challenges today call for teams of data experts to work together with data scientists to prepare for, analyze, and operationalize data problems.
Ultimately, what most organizations need is not more data scientists but a way to amplify their impact. On this front, Libby Duane Adams of Alteryx suggested that existing data scientists should divide their focus between macro insights and harnessing the collective expertise of existing analysts and business managers.
Don’t ignore your dark data
The explosion of data is arguably outpacing the ability of businesses to use it, culminating in dark data, which is information assets organizations collect, process, and store during regular business activities but generally fail to use for other purposes. It can uncover hidden correlations between pieces of information that were thought to be unrelated.
Dark data can represent a regulatory risk in some instances. For banks, regulators would not be impressed by the presence of existing data that revealed red flags of fraud or which could have been used to prevent a data breach.
According to Ajay Bhatia of Veritas, AI can be used to identify and manage untagged and unstructured data, quickly scanning, tagging, and classifying them for use. It can also be leveraged to parse vast amounts of data. Specifically, AI can easily manage the data volume to identify potential anomalies and uncover other hard-to-find insights.
Work to democratize your data
Silos and the inability to easily access relevant data are common bugbears of data teams and business users. They can impact data processing speeds and are vulnerable to rapid shifts in business requirements. Data democratization is often touted as a solution in these cases. But while it sounds easy, data democratization requires a fair amount of work to get the desired outcomes and often entails balancing availability, privacy, and security.
For one, data democratization does not mean making all data available to everyone, even if they are trusted internal users. A good example would be the prescription records of a healthcare organization – certain drugs might only be prescribed for unique conditions, and allowing general access would breach patient confidentiality.
According to Ram Thilak of Inchcape, the cloud is inextricably linked with data democratization. He said: “There is no better way for any organization to unlock that value and drive decision-making through data without cloud, and that is a no-brainer for anyone.”
Drive data from the top
Much as we like to, users may not gravitate towards data without an impetus from the top. According to Chong Yang Chan, managing director of Qlik for ASEAN, organizations need to drive analytics with a top-down approach by C-level executives who demonstrate clearly that they make decisions not with their “gut feelings”, but through data-backed.
“To encourage the organization to make use of a dashboard, for example, the CEO should ask for supporting evidence or data points from the dashboard before going ahead with a business recommendation,” said Chan.
So how can organizations gauge the state of their data science? Chan has a suggestion on how organizations can track their progress: “From the moment a problem is identified, how long does it take for stakeholders to gain the insights they need from the data?”
Paul Mah is the editor of DSAITrends. A former system administrator, programmer, and IT lecturer, he enjoys writing both code and prose. You can reach him at [email protected].
Image credit: iStockphoto/Jerome Maurice
Paul Mah
Paul Mah is the editor of DSAITrends, where he report on the latest developments in data science and AI. A former system administrator, programmer, and IT lecturer, he enjoys writing both code and prose.